Image
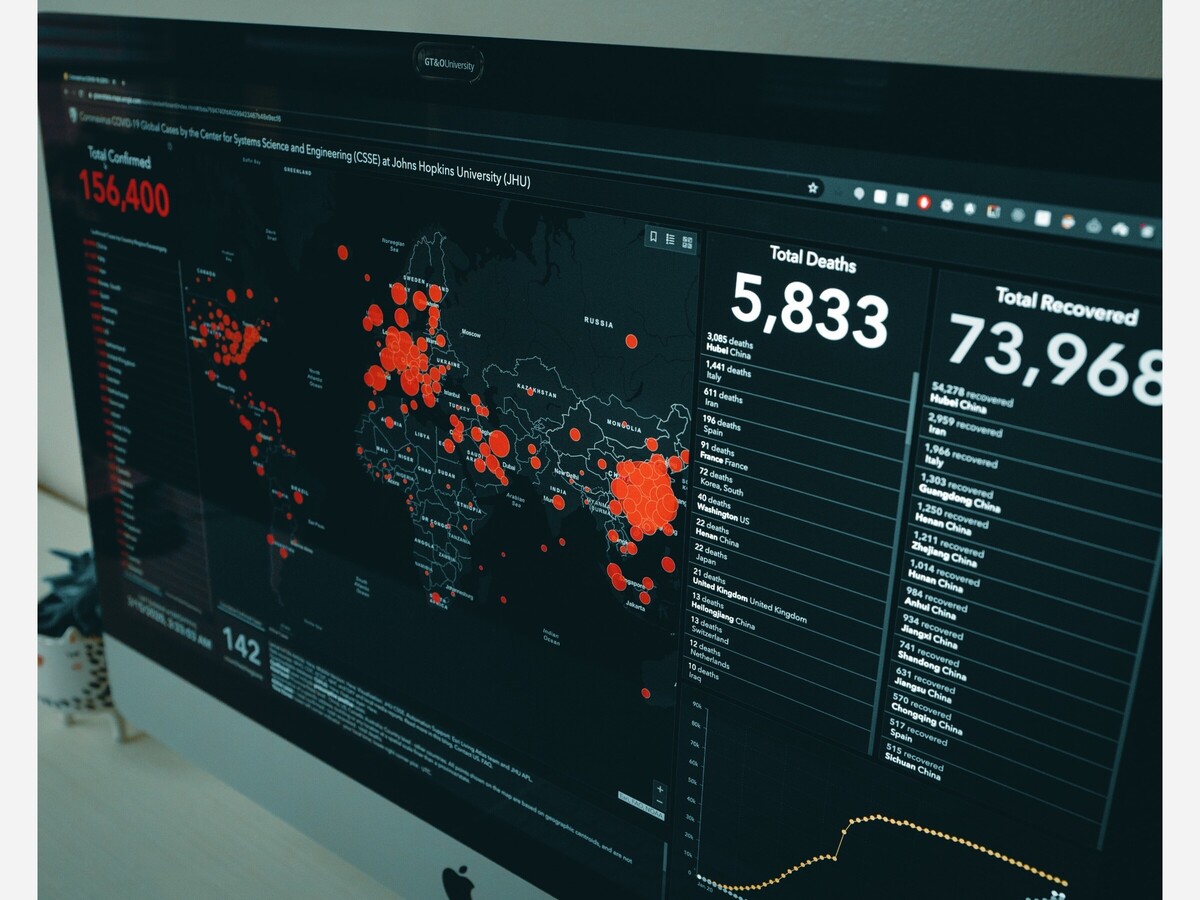
Statistics are a pervasive and influential force in modern society, as they provide a means for distilling complex information into digestible insights. They can shape public opinion, inform policy decisions, and guide scientific research. However, statistics can also be misleading, misused, or misunderstood. In this blog, we will explore the reality of statistics, why they can't always be relied upon for accurate information, and how to critically assess their validity.
Misleading Measures
One issue with relying on statistics for accurate information is that they can be misleading. For example, average is a widely used statistical measure prone to distortion by extreme values, known as outliers. When outliers are present, the standard may not accurately represent the central tendency of a dataset. The median or mode could be more appropriate in such cases, highlighting the importance of understanding the underlying data and selecting appropriate statistical measures.
Cherry-Picking and Confirmation Bias
Another challenge with statistics is that they can be cherry-picked to support a particular viewpoint or narrative. This selective use of data, known as confirmation bias, can lead to a distorted understanding of reality. Considering alternative explanations and examining multiple data sources is essential to understand a topic comprehensively.
Correlation vs. Causation
A common misconception is that correlation implies causation. However, correlation only indicates a relationship between variables, not suggesting that one causes the other. This misunderstanding can lead to faulty conclusions and misguided actions. Researchers must consider additional evidence, such as experimental studies, to establish causation and rule out potential confounding variables.
Sampling Bias
The accuracy of statistical information relies heavily on the representativeness of the samples used. Sampling bias occurs when a sample is not representative of the population it is meant to describe, leading to skewed results. Sampling bias includes nonresponse bias, where certain groups are less likely to respond to a survey, and selection bias, where the selection process influences the sample. Awareness of potential sampling biases is crucial when interpreting statistical information.
Data Manipulation and Misinterpretation
Statistics can also be manipulated intentionally or unintentionally to present a specific picture. This manipulation can involve using statistical techniques, omitting relevant data, or misinterpreting results. To guard against data manipulation and misinterpretation, it is essential to critically evaluate the source of the statistics, the methodology used, and the context in which they are presented.
Conclusion
While statistics can provide valuable insights and simplify complex information, they are not infallible. To ensure the accurate interpretation of statistical information, it is crucial to be aware of potential pitfalls, such as misleading measures, cherry-picking, correlation vs. causation, sampling bias, and data manipulation. By developing a critical mindset and understanding the limitations of statistics, we can make more informed decisions and avoid being misled by inaccurate or misused data.